AI v. Opioids: Fighting Back with Data
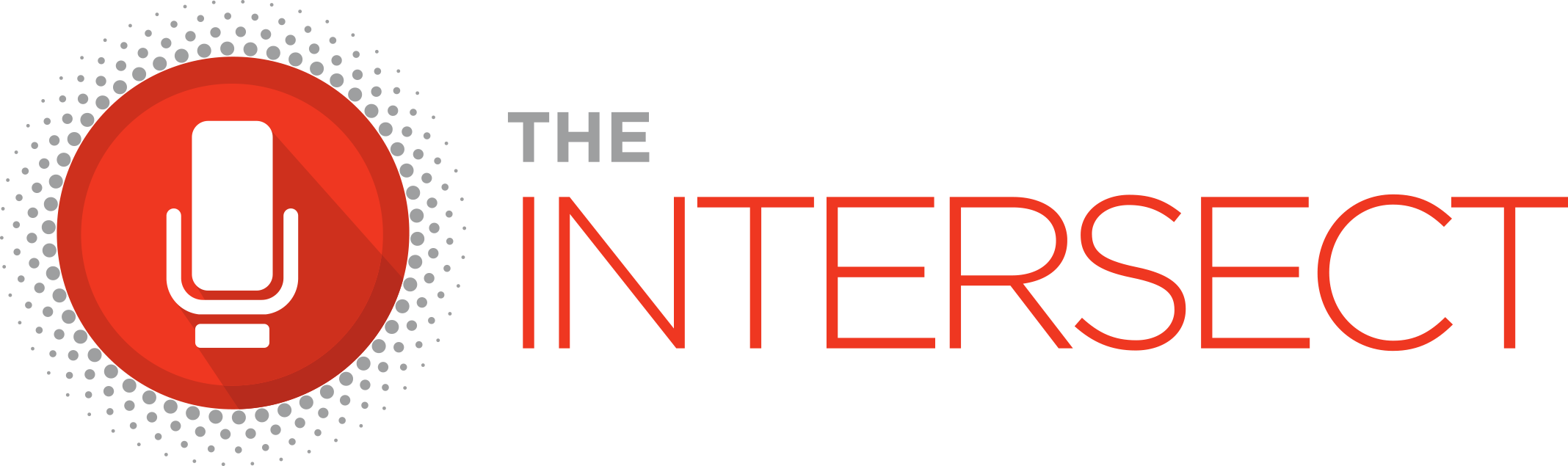
Challenges in areas ranging from education to the environment, gender to governance, health to housing don’t exist in a vacuum. Each month, Abt experts from two disciplines will explore ideas for tackling these challenges in our new monthly podcast, The Intersect. Sign up for monthly e-mail notifications here. Catch up with previous episodes here.
At the outset of the opioid crisis, experts didn’t know what to look for. As our mastery of AI and machine learning grows we can marry it to our deeper understanding of this epidemic and use it to create solutions. Take 15 minutes to listen to Abt experts Dana Hunt and Gabriel Krieshok in this episode of The Intersect:
Read the Transcript:
Eric Tischler: Hi and welcome to the Intersect. Today I'm joined by Dana Hunt and Gabriel Krieshok.
Dana is a principal scientist here at Abt. She’s a highly regarded expert on crime, substance abuse, illegal drug use and drug treatment, and co-writer of the recent blog titled “Should We Have Seen the Opioid Crisis Coming?”
Gabriel is a data scientist who manages the diverse portfolio of Information and Communication Technology for Development, ranging from global health to agriculture to education across middle and low income countries.
Thank you both for joining me.
Dana Hunt and Gabriel Krieshok: Thank you for having us.
Eric: So let's talk about that blog, Dana—“Should We Have Seen the Opioid Crisis Coming?”
Dana Hunt: Sure. I wrote that originally because this was something—I should back up a little bit. You know there have been lots of cycles of rising and falling heroin use and opioid use since I've been doing this, which is an awfully long time. And one of the things that you look for in any substance abuse change or epidemic is when that use which hadn't been particularly there before is now in places where it hadn't appeared. And also in groups in which it's not yet appeared. The young—people who were 18 to 22 are the replacements for old users who move out of drug use, particularly something like opioids. And when you see a rise in the use among the 18 to 22 year olds in a particular drug and it is a rise that's significantly higher than what you would expect from the past trends that's telling you that something is happening in either availability of the drug but certainly in the use pattern of the drug. That's exactly what we saw starting in 2007 and actually some of the other data sources that we looked at saw it in 2005, where there was a dramatic increase—in this case a project that I ran for years that is looked at arrestees in cities across the country—and you saw in cities like Charlotte, Indianapolis, Indiana, Denver, places that are not characteristically, historically ones that have had a heroin problem.
Eric: So, were we not looking in the right places?
Dana: The thing is I think we were, you know, as a drug policy group we weren't really looking for heroin and we weren't looking for the prescription opiates yet, which are directly related to the rise of heroin use.
We were looking at marijuana. We were looking at other drugs but we weren't looking there and we weren't really looking for those two indicators, which is increased use among the young and increased use in places where you don't see it. If we see a 4 or 5 percent use in New York City, that doesn't mean a great deal; if you see a 10 percent use in Indianapolis, that means a great deal. The dataset that that we collected over the many years are the same population; it is people within 48 hours of arrest and they’re drug tested. It's not a self report, it's a clear indication of what was in their system at the time of arrest.
Eric: So, Gabriel, what could we have done using AI, using machine learning to alert ourselves to what was going on? Is there something we could be doing now to avoid similar or unexpected crises?
Gabriel Krieshok: I think Dana kind of hit it on the head in terms of there's a lot of data out there, right? And so I think one of the big challenges that we've seen in the past—and currently—is just: How do we think about putting all this data together and then finding the patterns in it?
Dana: Right.
Gabriel: And, thankfully, that's one of the things that we see or we're starting to see in machine learning and AI is that, you know, you're kind of voracious about data. It really wants to find patterns and match patterns. And so, if we know the kinds of questions that we want to ask and we know what we're looking for, we can give it a jumpstart and sort of have an idea of what we want to look for but also, as we get a little bit more sophisticated, we can start to see patterns and things pop out of us that we might not have seen before.
Dana: I think that's exactly right, Gabriel. You know, if you had looked at what's called the TED data, the treatment episode dataset which is everybody who is admitted to public and publicly funded treatment programs in the country starting in 2005, you saw a massive increase between 2005 and 2015 for heroin admissions, a 54 percent increase, up to almost a half a million people.
Eric: So this gets back to what Gabriel said about knowing to ask the right questions so we can find those blind spots. Dana, what questions do we need to be asking ourselves and do we need to be feeding into A.I. to identify those blind spots?
Dana: I think one of the questions that needs to be put out there is that—I don't know if it's a question but it's a clear understanding that drug use is regional and that looking at national estimates is not necessarily the way to go to figure out these patterns.
An example I use quite frequently is methamphetamine. In our 2013-2014 collection of these data, 52 percent of the guys who were arrested in Sacramento tested positive for methamphetamine; less than 2 percent on the East Coast. If you smush those two together to try to find a pattern it wouldn't tell you very much; it wouldn't tell you about what's really happening. So the first question is can we gather some of these regional data to figure out what's happening and put them together. I think the idea of blending data sources from treatment, from the criminal justice data, from availability data, from what's called the heroin domestic monitoring program that the DEA does—all of those sources, put out regionally, can tell you a great deal about what's happening.
Gabriel: I was just going to say, you know, my work coming from the more international sector and applying data science—and some of the machine learning projects and products that we're working on—makes me think that a lot of what we do is spend time really trying to understand the data itself. And trying to understand the limitations that we're currently working with and the kinds of biases—the inherent biases—that are already in the datasets that we're looking at and spending just, frankly, a lot of time—you know 80 percent of the time, basically doing data cleaning and things like that. I'm curious, from your perspective, how you sort of characterize the datasets that you were just talking about and if they're at that point of maturity where we're ready to start really putting them together and trying to understand things, so that we could then take that next step and apply things like machine learning and finding those patterns.
Dana: Some of these data sets are at that point, certainly ones that the Substance Abuse and Mental Health Services Administration have been collecting for decades. The TEDs data is at that point because it's collected at intake. If you receive federal money for substance abuse treatment—if you're a program—you have to do this intake data and you have to turn it in to your state, the state turns it in to the Feds. That is a data set that is mature enough to be looked at in this way.
Some of the criminal justice data which we contain—we have a lot here at Abt that we work with—may be not quite as ready, but I think I think this is definitely an area in which A.I. and machine learning could be very helpful in trying to figure out these patterns. Even going to, you know, scraping data on the web because there's a great deal of information out there that's, like, crowdsourcing kinds of information—not clean, not very good but helpful with these other datasets that are there. The DEA collects a great deal of data that systematically could be used.
Eric: If the use is increasing and we've seen the mistakes we made with opioid use, you know, in 2005, what can we be doing now if heroin cocaine use is still on the rise?
Dana: I think that the kinds of things that are putting these data sets together and looking at them together including, things like availability, things like what's called price per pure gram—that's a very important item in terms of what is happening with cocaine right now. When the price for a pure gram of the drug rises or falls, that tells you something about the availability of the drug. When you have very pure—which we did with heroin, very pure heroin on the street—but the price has dropped, that's telling you something about that aspect of the whole equation, which is the availability and the marketing and the pushing—the pushing the drug into new areas, into West Virginia, you know. The states with the highest increases of heroin deaths. Right now, West Virginia and Ohio are there.
Gabriel: Dana, you know, the work that we do in the data science division that I'm a part of is that we do some percentage—let's say 50 percent—of research tasks. You know, we're sort of looking in hindsight and sort of saying, you know, kind of in the way that you're describing a little bit “What are the numbers showing what's happened?” and “How do we characterize what happened?” But then let's say the other 50 percent of what we do is more forward looking, sort of like project implementation. And so it might be the case where we want to, you know, position ourselves in such a way to build a project or to be part of some program that basically says, “OK ,you know, as this data comes in or as we understand something let's try and mitigate this or let's use this as sort of a monitoring tool that allows us to understand these quick feedback loops, to be able to change something on the ground or to mobilize resources quickly.”
So, I'm curious, from your perspective, what sorts of things might be—you know, talking about machine learning and A.I. in the past tense, we can analyze and find those patterns. I'm wondering if there are opportunities to think about it looking forward, like maybe we can use it to mobilize resources differently or distribute efforts based on regional hotspots or things like that.
Dana: Absolutely, it could be used for that. There's a great deal that's known about patterns of drug use by doing just what you said: looking backwards. We can use some of those things to look forward. Just on preparation level: had we seen or had we paid attention to the fact that heroine was rising in some of these places we would have decided to look at methadone as a treatment.
Methadone has—as you know, the bottom fell out of the opportunity for methadone in states. There are very, very few programs anymore. That should have been a heads up that says “We're going to need this.” We're looking at it now with cocaine. Cocaine is rising again: the purity is high, the price is low. All of the indicators are that the use is high and distribution is high again. There's not a lot of cocaine treatment either. That would be something going forward that you could look at.
Gabriel: I'm curious—I have another question, if I’m allowed …
Eric: Please!
Gabriel: One of the things—like just what you just said in terms of cocaine treatment—we always think about the A.I. piece as identifying these patterns, I'm curious if we can identify things that would have an influence or impact on behavior change. So, you’re not just looking at statistics but saying you know certain people in certain conditions respond differently or behave differently towards different incentives or drug treatments or things like that. Have you given that much thought, in terms of how we can distribute our resources and then say, you know, “For X, Y, Z person and such and such sort of situation, it looks like, based on the data and based on our sort of algorithm that we have, that this would be the most appropriate sort of treatment response” or anything like that?
Dana: You know, that's a whole field as to what is effective for different kinds of treatment but it certainly would be something that you'd want to start projecting forward and gearing up.
Gabriel: Right. Right, right, right.
Dana: I think that the task is putting these pieces together and using data science to project forward to see based on patterns in the past—because we have long histories of patterns in the past—and to see what the expectation would be and what does that mean for developing treatment, developing prevention materials, developing hotspot policing, you know, all kinds of things that could be addressed if we looked forward.
Gabriel, I would love to sit down with you and do this. Figure out how we can do something with it.
Gabriel: I would too. We'll go off line to do it in a secret intersect.
Eric: And that's an even better ending, so thank you both for joining me!